[edit]
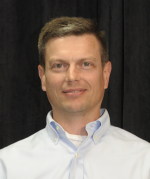
Eric Brown
Research Scientist, Watson Technologies
Thomas J. Watson Research Center
The Jeopardy! Challenge and Beyond
Abstract:
Watson, named after IBM founder Thomas J. Watson, was built by a team of IBM researchers who set out to accomplish a grand challenge---build a computing system that rivals a human's ability to answer questions posed in natural language with speed, accuracy and confidence. The quiz show Jeopardy! provided the ultimate test of this technology because the game's clues involve analyzing subtle meaning, irony, riddles and other complexities of natural language in which humans excel and computers traditionally fail. Watson passed its first test on Jeopardy!, beating the show's two greatest champions in a televised exhibition match, but the real test will be in applying the underlying natural language processing and analytics technology in business and across industries. In this talk I will introduce the Jeopardy! grand challenge, present an overview of Watson and the DeepQA technology upon which Watson is built, and explore future applications of this technology.
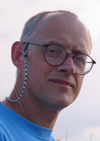
Christian Robert
Professor, Ceremade
University of Paris - Dauphine
Approximative Bayesian Computation (ABC): computational advances versus
inferential uncertainties
Abstract:
The lack of closed form likelihoods has been the bane of Bayesian
computation for many years and, prior to the introduction of MCMC methods,
a strong impediment to the propagation of the Bayesian paradigm. We are
now facing models where an MCMC completion of the model towards
closed-form likelihoods seems itself unachievable and where a further
degree of approximation appears to be unavoidable. In this review talk, I
will present the motivation for approximative Bayesian computation (ABC)
methods, the various implementations found in the current literature, as
well as the inferential, rather than computational, challenges set by
these methods and not completely solved at this stage.
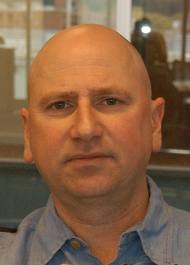
Larry Wasserman
Professor, Department of Statistics
Carnegie Mellon University
Geometric and Topological Inference
Abstract: I will discuss the problem of inferring geometric and topological features of point clouds and functions. Examples include: estimating clusters and manifolds, filaments detection, estimating the homology groups of a manifold, and ridge estimation. In each case I'll discuss the estimation methods and minimax rates.