[edit]
AISTATS*2010 Invited Speakers
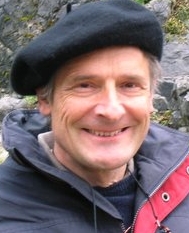
Richard Gill
Mathematical Institute, Leiden University
Forensic Statistics: Where Are We and Where are We Going?I will discuss the present situation of Forensic statistics. Rapid developments in forensic science are putting statistics and probability more and more into the court-room lime-light, often with apalling results. Why is this and where should we go? Standard Bayesian and standard frequentist statistics are based on the wrong paradigms. Forensic statisticians have to learn from the learning community. But in forensic statistics, N=1. How can we learn?
Bio: Richard Gill has occupied the Chair of Mathematical Statistics at the University of Leiden since 2006, having held other posts in the Netherlands since 1974. His research interests are in law, statistics in law, biostatistics, genetics, survival analysis, semiparametric models, causality, machine learning, statistical image analysis and quantum statistical information. He is fascinated by foundational aspects of statistics, probability and quantum physics, and by the societal role of science in general and of statistics in particular.
In his work on quantum information he applies ideas and methodology from probability and statistics to experimental and technological problems in quantum science. In the new biosciences he sees fantastic challenges for probability and statistics, and he is especially interested in statistical problems of forensic DNA profiling.
He is an elected member of the Dutch Royal Academy of Sciences, he
is currently president of the Dutch Society for Statistics and
Operations Research, and he holds the Distinguished Lorentz Fellowship
for 2010-2011 for his contributions to forensic statistics. During
2007-2010 he was deeply involved in the movement to have the case of
Lucia de Berk reopened, fighting a miscarriage of justice that was
righted in April, 2010, as widely reported in the international press.
John Lafferty
School of Computer Science, Carnegie Mellon University
Nonparametric Learning of Functions and Graphs in High DimensionsWe present recent work on several nonparametric learning problems in the high dimensional setting. In particular, we present theory and methods for estimating sparse regression functions, additive models, and graphical models. For additive models, we present a functional version of methods based on l1 regularization for linear models. For graphical models, we develop methods for estimating the underlying graph based only on observations. One approach is something we call "the nonparanormal," which uses copula methods to transform the variables by nonparametric functions, relaxing the strong distributional assumptions made by the Gaussian graphical model. Another approach is to restrict the family of allowed graphs to spanning forests, enabling the use of fully nonparametric density estimation. All of the approaches are easy to understand, simple to use, theoretically well supported, and effective for modeling of high dimensional data. Joint work with Anupam Gupta, Han Liu, Larry Wasserman, and Min Xu.
Bio: John Lafferty is a professor in the Computer Science Department and the Machine Learning Department within the School of Computer Science at Carnegie Mellon University, where he also holds a joint appointment in the Department of Statistics. His Ph.D. is in Mathematics from Princeton University, where he was part of the Program in Applied and Computational Mathematics. Before joining CMU he was a Research Staff Member at the IBM Watson Research Center in Yorktown Heights, New York, where he first starting working at the interface of AI and Statistics. His recent research interests are in text analysis, machine learning, and statistical learning theory, with a recent focus on nonparametric methods for high dimensional data. He has served as co-director of CMU's Ph.D. Program in Machine Learning, and was recently paroled after serving a term as program co-chair of the 2009 NIPS Conference.
Simon Tavaré
Department of Applied Mathematics and Theoretical Physics, and Department of Oncology, University of Cambridge & Program in Molecular and Computational Biology, USC
Approximate Bayesian Computation: What, Why and How?Approximate Bayesian Computation (ABC) arose in response to the difficulty of simulating observations from posterior distributions determined by intractable likelihoods. The method exploits the fact that while likelihoods may be impossible to compute in complex probability models, it is often easy to simulate observations from them. ABC in its simplest form proceeds as follows: (i) simulate a parameter from the prior; (ii) simulate observations from the model with this parameter; (iii) accept the parameter if the simulated observations are close enough to the observed data. The magic, and the source of potential disasters, is in step (iii). This talk will outline what we know (and don't!) about ABC and illustrate the methods with applications to the fossil record and stem cell biology.
Bio: Simon Tavaré has for many years worked on statistical problems arising in molecular biology, human genetics, population genetics, molecular evolution bioinformatics and computational biology. Among his methodological interests is stochastic computation, including ABC, the topic of his lecture.
He is a Professor in the Department of Applied Mathematics and Theoretical Physics and Professor of Cancer Research (Bioinformatics) in the Oncology Department at the University of Cambridge. He is also a Senior Group Leader in the new Cancer Research UK Cambridge Research Institute. His group there focuses mainly on cancer genomics and evolutionary approaches to cancer. In 2009 he was elected a Fellow of the Academy of Medical Sciences.
Simon is also a Research Professor, and George and Louise Kawamoto Chair in Biological Sciences, at the University of Southern California. He is PI of the NIH Center of Excellence in Genomic Science at USC, which is developing computational and experimental approaches for understanding how genotype relates to phenotype.