[edit]
Invited Speakers
Speaker Name: Profressor David Blei, Columbia University
Talk Title: Black Box Variational Inference and Deep Exponential Families
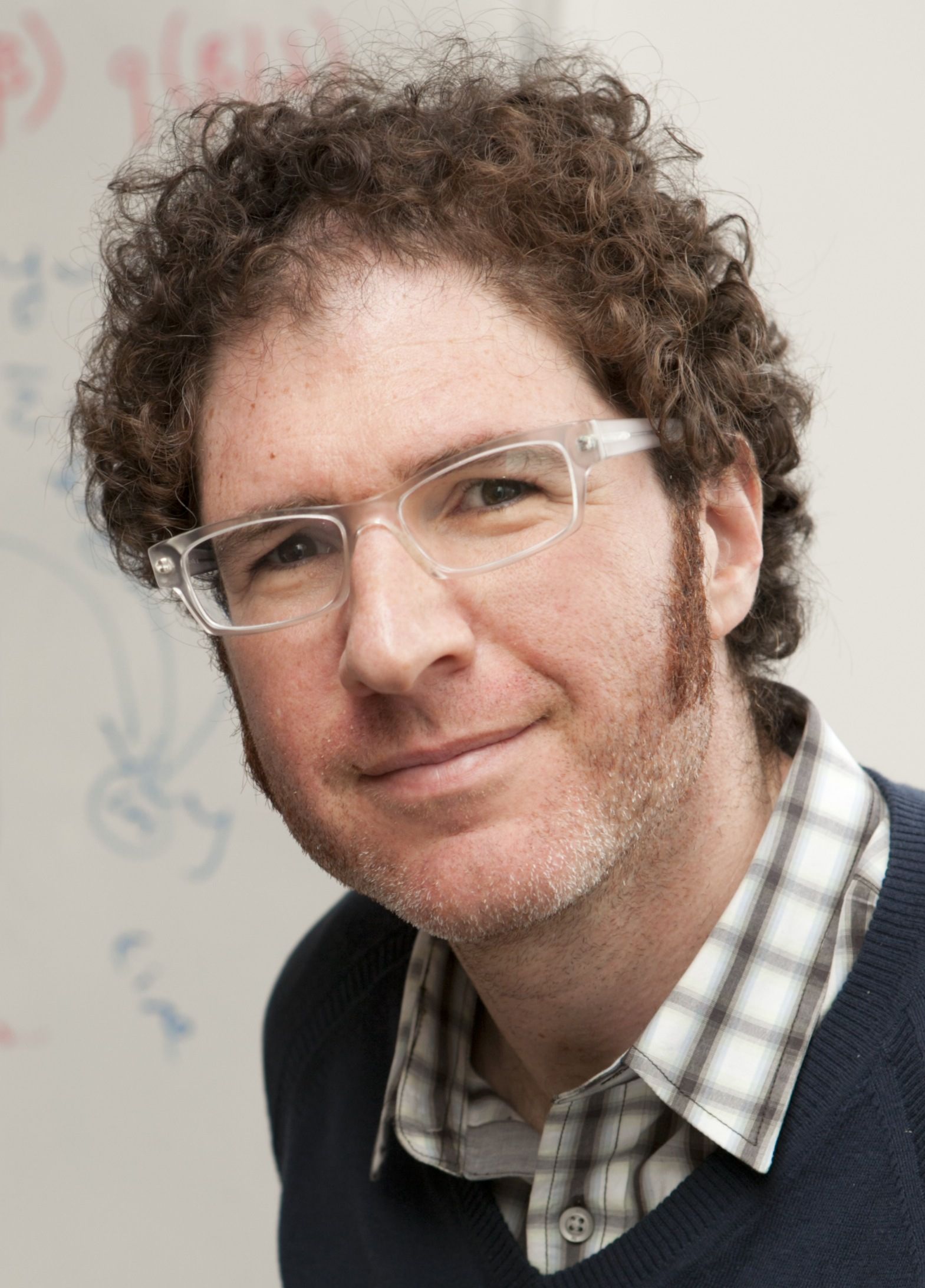
Biography:
David Blei is a Professor of Statistics and Computer Science at Columbia University, and a member of the Columbia Data Science Institute. His research is in statistical machine learning, involving probabilistic topic models, Bayesian nonparametric methods, and approximate posterior inference with massive data. He works on a variety of applications, such as text, images, music, social networks, user behavior, and scientific data. David has received several awards for his research, including a Sloan Fellowship (2010), Office of Naval Research Young Investigator Award (2011), Presidential Early Career Award for Scientists and Engineers (2011), Blavatnik Faculty Award (2013), ACM-Infosys Foundation Award (2013), and a Guggenheim fellowship. He is a fellow of the ACM and the IMS.
Speaker Name: Professor Jennifer Hill, NYU Steinhardt
Talk Title: Causal inference at the intersection of machine learning and statistics: opportunities and challenges
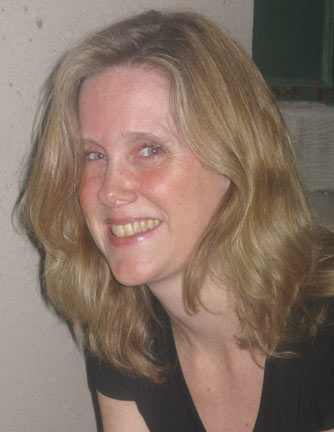
Biography:
Jennifer Hill works on development of methods that help us to answer the causal question that are so vital to policy research and scientific development. In particular she focuses on situations in which it is difficult or impossible to perform traditional randomized experiments, or when even seemingly pristine study designs are complicated by missing data or hierarchically structured data. Most recently Hill has been pursuing two major strands of research. The first focuses on Bayesian nonparametric methods that allow for flexible estimation of causal models without the need for methods such as propensity score matching. The second line of work pursues strategies for exploring the impact of violations of typical assumptions in this work that require that all confounders have been measured. Hill has published in a variety of leading journals including Journal of the American Statistical Association, American Political Science Review, American Journal of Public Health, and Developmental Psychology. Hill earned her PhD in Statistics at Harvard University in 2000 and completed a post-doctoral fellowship in Child and Family Policy at Columbia University's School of Social Work in 2002. Hill is also the Co-Director of the Center for Research Involving Innovative Statistical Methodology (PRIISM) and Co-Director of and the Master of Science Program in Applied Statistics for Social Science Research (A3SR).
Speaker Name: Professor Andreas Krause, ETH Zurich
Talk Title: Towards Safe Reinforcement Learning
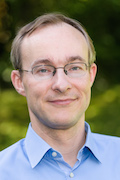
Biography:
Andreas Krause is a Professor of Computer Science at ETH Zurich, where he leads the Learning & Adaptive Systems Group. He also serves as Academic Co-Director of the Swiss Data Science Center. Before that he was an Assistant Professor of Computer Science at Caltech. He received his Ph.D. in Computer Science from Carnegie Mellon University (2008) and his Diplom in Computer Science and Mathematics from the Technical University of Munich, Germany (2004). He is a Microsoft Research Faculty Fellow, received an ERC Starting Investigator grant, the German Pattern Recognition Award, the Okawa Foundation Research Grant recognizing top young researchers in telecommunications as well as the ETH Golden Owl teaching award. His research on machine learning and adaptive systems has received awards at several premier conferences and journals.