[edit]
AISTATS*2014 Poster Sessions
Poster Session 1, Tuesday, 22 April
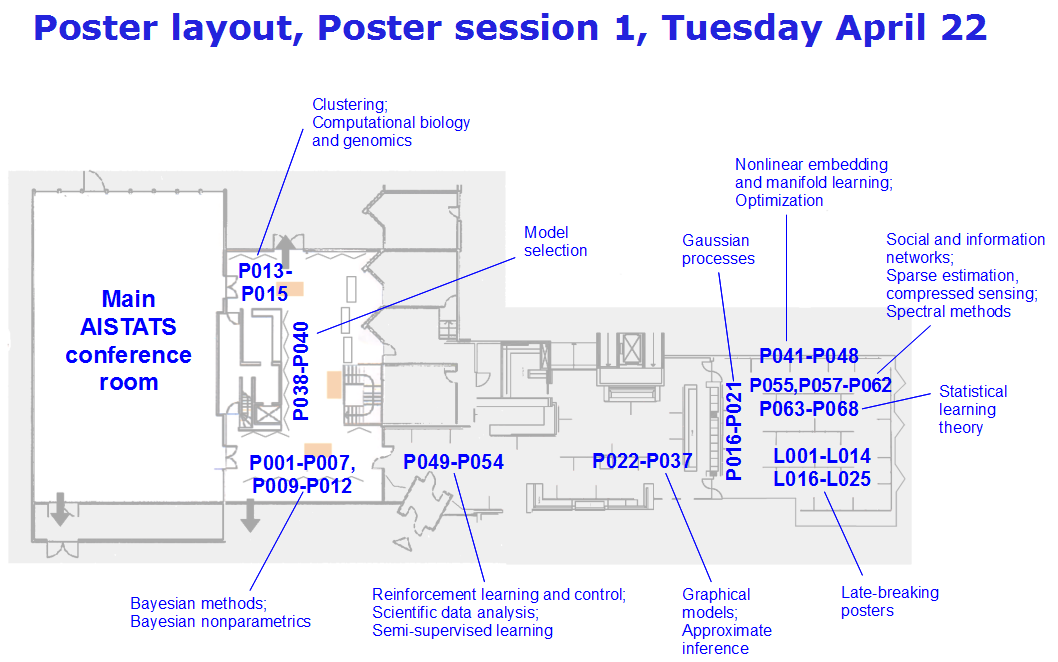
Click the image to view the layout in larger size.
Bayesian methods
P001 Sequential crowdsourced labeling as an epsilon-greedy exploration in a Markov Decision Process
Vikas Raykar; Priyanka Agrawal
P002 Active Boundary Annotation using Random MAP Perturbations
Subhransu Maji; Tamir Hazan; Tommi Jaakkola
P003 Bayesian Switching Interaction Analysis Under Uncertainty
Zoran Dzunic; John Fisher III
Notable paper: P004 Analysis of Empirical MAP and Empirical Partially Bayes: Can They be Alternatives to Variational Bayes?
Shinichi Nakajima; Masashi Sugiyama
P005 SMERED: A Bayesian Approach to Graphical Record Linkage and De-duplication
Rebecca Steorts; Rob Hall; Stephen Fienberg
Bayesian nonparametrics
P006 Bayesian Nonparametric Poisson Factorization for Recommendation Systems
Prem Gopalan; Francisco J. Ruiz; Rajesh Ranganath; David Blei
P007 Incremental Tree-Based Inference with Dependent Normalized Random Measures
Juho Lee; Seungjin Choi
P009 Student-t Processes as Alternatives to Gaussian Processes
Amar Shah; Andrew Wilson; Zoubin Ghahramani
P010 Bayesian Logistic Gaussian Process Models for Dynamic Networks
Daniele Durante; David Dunson
P011 The Dependent Dirichlet Process Mixture of Objects for Detection-free Tracking and Object Modeling
Willie Neiswanger; Frank Wood; Eric Xing
P012 Pan-sharpening with a Bayesian nonparametric dictionary learning model
Xinghao Ding; Yiyong Jiang; Yue Huang; John Paisley
Clustering
P013 Connected Sub-graph Detection
Jing Qian; Venkatesh Saligrama; Yuting Chen
Computational biology and genomics
P014 Sparse Bayesian Variable Selection for the Identification of Antigenic Variability in the Foot-and-Mouth Disease Virus
Vinny Davies; Richard Reeve; William Harvey; Francois Maree; Dirk Husmeier
P015 Context Aware Group Nearest Shrunken Centroids in Large-Scale Genomic Studies
Juemin Yang; Fang Han; Rafael Irizarry; Han Liu,
Gaussian processes
P016 Hybrid Discriminative-Generative Approach with Gaussian Processes
Ricardo Andrade Pacheco; James Hensman; Max Zwiessele; Neil Lawrence
P017 Analytic Long-Term Forecasting with Periodic Gaussian Processes
Nooshin HajiGhassemi; Marc Deisenroth,
P018 Tilted Variational Bayes
James Hensman; Max Zwiessele; Neil Lawrence
P019 Bat Call Identification with Gaussian Process Multinomial Probit Regression and a Dynamic Time Warping Kernel
Vassilios Stathopoulos; Veronica Zamora-Gutierrez; Kate Jones; Mark Girolami
P020 Explicit Link Between Periodic Covariance Functions and State Space Models
Arno Solin; Simo Särkkä
P021 Probabilistic Solutions to Differential Equations and their Application to Riemannian Statistics
Philipp Hennig; Søren Hauberg
Graphical models and approximate inference
P022 Learning Heterogeneous Hidden Markov Random Fields
Jie Liu; Chunming Zhang; Elizabeth Burnside; David Page
P023 Random Bayesian networks with bounded indegree
Eunice Yuh-Jie Chen; Judea Pearl
P024 Active Learning for Undirected Graphical Model Selection
Divyanshu Vats; Robert Nowak; Richard Baraniuk
P025 Nonparametric estimation and testing of exchangeable graph models
Justin Yang; Christina Han; Edoardo Airoldi
P026 An inclusion optimal algorithm for chain graph structure learning
Jose Peña; Dag Sonntag; Jens Nielsen
P027 Joint Structure Learning of Multiple Non-Exchangeable Networks
Chris Oates; Sach Mukherjee
P028 Adaptive Variable Clustering in Gaussian Graphical Models
Siqi Sun; Yuancheng Zhu; Jinbo Xu
P029 On the Testability of Models with Missing Data
Karthika Mohan; Judea Pearl
P030 Interpretable Sparse High-Order Boltzmann Machines
Martin Renqiang Min; Xia Ning; Chao Cheng
P031 Mixed Graphical Models via Exponential Families
Eunho Yang; Yulia Baker; Pradeep Ravikumar; Genevera Allen; Zhandong Liu
P032 Learning Bounded Tree-width Bayesian Networks using Integer Linear Programming
Pekka Parviainen; Hossein Shahrabi Farahani; Jens Lagergren
P033 Learning Optimal Bounded Treewidth Bayesian Networks via Maximum Satisfiability
Jeremias Berg; Matti Järvisalo; Brandon Malone
P034 Exploiting the Limits of Structure Learning via Inherent Symmetry
Peng He; Changshui Zhang
P035 A Gaussian Latent Variable Model for Large Margin Classification of Labeled and Unlabeled Data
Do-kyum Kim; Matthew Der; Lawrence Saul
P036 Learning with Maximum A-Posteriori Perturbation Models
Andreea Gane; Tamir Hazan; Tommi Jaakkola
P037 Efficient Inference for Complex Queries on Complex Distributions
Lili Dworkin; Michael Kearns; Lirong Xia
Model selection
P038 Robust learning of inhomogeneous PMMs
Ralf Eggeling; Teemu Roos; Petri Myllymäki; Ivo Grosse
P039 Fully-Automatic Bayesian Piecewise Sparse Linear Models
Riki Eto; Ryohei Fujimaki; Satoshi Morinaga; Hiroshi Tamano
P040 Efficient Transfer Learning Method for Automatic Hyperparameter Tuning
Dani Yogatama; Gideon Mann
Nonlinear embedding and manifold learning
P041 Linear-time training of nonlinear low-dimensional embeddings
Max Vladymyrov; Miguel Carreira-Perpinan
P042 Sketching the Support of a Probability Measure
Joachim Giesen; Soeren Laue; Lars Kuehne
Optimization
P043 Lifted MAP Inference for Markov Logic Networks
Somdeb Sarkhel; Deepak Venugopal; Parag Singla; Vibhav Gogate
P044 A Stepwise uncertainty reduction approach to constrained global optimization
Victor Picheny
P045 Global Optimization Methods for Extended Fisher Discriminant Analysis
Satoru Iwata; Yuji Nakatsukasa; Akiko Takeda
P046 Efficient Low-Rank Stochastic Gradient Descent Methods for Solving Semidefinite Programs
Jianhui Chen; Tianbao Yang; Shenghuo Zhu
P047 Distributed optimization of deeply nested systems
Miguel Carreira-Perpinan; Weiran Wang
P048 Accelerated Stochastic Gradient Method for Composite Regularization
Wenliang Zhong; James Kwok
Reinforcement learning and control
P049 Dynamic Resource Allocation for Optimizing Population Diffusion
Shan Xue; Alan Fern; Daniel Sheldon
P050 Optimality of Thompson Sampling for Gaussian Bandits Depends on Priors
Junya Honda; Akimichi Takemura
Scientific data analysis
P051 A Statistical Model for Event Sequence Data
Kevin Heins; Hal Stern
P052 Towards building a Crowd-Sourced Sky Map
Dustin Lang; David Hogg; Bernhard Schölkopf
Semi-supervised learning
P053 Class Proportion Estimation with Application to Multiclass Anomaly Rejection
Tyler Sanderson; Clayton Scott
P054 Heterogeneous Domain Adaptation for Multiple Classes
Joey Tianyi Zhou; Ivor W.Tsang; Sinno Jialin Pan; Mingkui Tan
Social and information networks
P055 Computational Education using Latent Structured Prediction
Tanja Käser; Alexander Schwing; Tamir Hazan; Markus Gross
Sparse estimation, compressed sensing
P057 Path Thresholding: Asymptotically Tuning-Free High-Dimensional Sparse Regression
Divyanshu Vats; Richard Baraniuk
P058 FuSSO: Functional Shrinkage and Selection Operator
Junier Oliva; Barnabas Poczos; Timothy Verstynen; Aarti Singh; Jeff Schneider; Fang-Cheng Yeh; Wen-Yih Tseng
P059 Information-Theoretic Characterization of Sparse Recovery
Cem Aksoylar; Venkatesh Saligrama
P060 Average Case Analysis of High-Dimensional Block-Sparse Recovery and Regression for Arbitrary Designs
Waheed Bajwa; Marco Duarte; Robert Calderbank
Spectral methods
P061 High-Dimensional Density Ratio Estimation with Extensions to Approximate Likelihood Computation
Rafael Izbicki; Ann Lee; Chad Schafer
P062 Low-Rank Spectral Learning
Alex Kulesza; N. Raj Rao; Satinder Singh
Statistical learning theory
P063 Tight Bounds for the Expected Risk of Linear Classifiers and PAC-Bayes Finite-Sample Guarantees
Jean Honorio; Tommi Jaakkola
P064 Decontamination of Mutually Contaminated Models
Gilles Blanchard; Clayton Scott
P065 A Finite-Sample Generalization Bound for Semiparametric Regression: Partially Linear Models
Ruitong Huang; Csaba Szepesvari
P066 New Bounds on Compressive Linear Least Squares Regression
Ata Kaban
P067 PAC-Bayesian Collective Stability
Ben London; Bert Huang; Ben Taskar; Lise Getoor
P068 PAC-Bayesian Theory for Transductive Learning
Luc Bégin; Pascal Germain; François Laviolette; Jean-Francis Roy
Late-breaking Posters
L001 VARIOUS GOODNESS-OF-FIT TESTS BASED ON THE PROBABILITY WEIGHTED EMPIRICAL CHARACTERISTIC FUNCTIONLeonard Santana; James Allison; Simos Meintanis
L002 Dependent Pairs of Maximum Mean Descrepancy Tests
Ioannis Antonoglou; Matthew Blaschko; Arthur Gretton,
L003 Ensembles of limited dependence Bayesian Classifiers for Protein-Protein Interaction prediction from Sequence Data
Jana Kludas; Juho Rousu
L004 Approximate Models and Robust Decisions
James Watson; Chris Holmes
L005 Decision-theoretic justifications for Bayesian hypothesis testing using credible sets
Måns Thulin
L006 Bayesian nonparametric regression via probabilistic ranking models
Tristan Gray-Davies; Chris Holmes; Francois Caron
L007 A parameter-free clustering prior based on partition entropy
Fritz Obermeyer; Jonathan Glidden
L008 A performance comparison of generative and discriminative models in causal and anticausal problems
Patrick Blöbaum; Shohei Shimizu; Takashi Washio
L009 Improving Direct Contact Analysis in the Third Dimension
Marcin Skwark; Christoph Feinauer
L010 Joint Detection of Multiple Causal Disease Variants
Ingileif Hallgrimsdottir; Brielin Brown
L011 Trainable COSFIRE Filters for keypoint detection, object localization, and pattern recognition
George Azzopardi; Nicolai Petkov
L012 Opening the way for deep Gaussian processes on massive data
Andreas Damianou; James Hensman; Neil Lawrence
L013 Uniform random generation of large acyclic digraphs
Jack Kuipers; Giusi Moffa
L014 Reconstruction quality of a biological network when its constituting elements are partially observed
Victor Picheny; Jimmy Vandel; Matthieu Vignes; Nathalie Villa-Vialaneix
L016 Distributed parameter estimation of discrete hierarchical models via marginal likelihoods
Helene Massam; Nanwei Wang
L017 Distributed Coordinate Descent for L1-regularized Logistic Regression
Ilya Trofimov; Alexander Genkin
L018 A Problem with the Use of Cross-Validation for Selecting among Multilevel Models
Wei Wang; Andrew Gelman
L019 A Bayesian Approach to Generalized Ensemble Markov Chain Monte Carlo
Jes Frellsen; Ole Winther; Jesper Ferkinghoff-Borg
L020 The Role of Dimensionality Reduction in Linear Classification
Weiran Wang; Miguel Carreira-Perpinan
L021 Generative Artificial Neural Networks (GANNs) for nonlinear regression with prediction uncertainty. Application to astrophysical parameter estimation using Gaia RVS spectra
Diego Fustes Villadóniga; Minia Manteiga; Carlos Dafonte; Ana Ulla
L022 LASS: A Simple Assignment Model with Laplacian Smoothing
Miguel Carreira-Perpinan; Weiran Wang
L023 A Generalized Method of Moments Algorithm for Learning Probabilistic Context-Free Grammars
Gabi Teodoru
L024 New Guarantees for Alternating Minimization
Sujay Sanghavi
L025 A diagnostic model for autoimmune disease
Guðný Árnadóttir; Dagrún Jónasdóttir; Björn Lúðvíksson; Bjarni Halldórsson
Poster Session 2, Thursday, 24 April
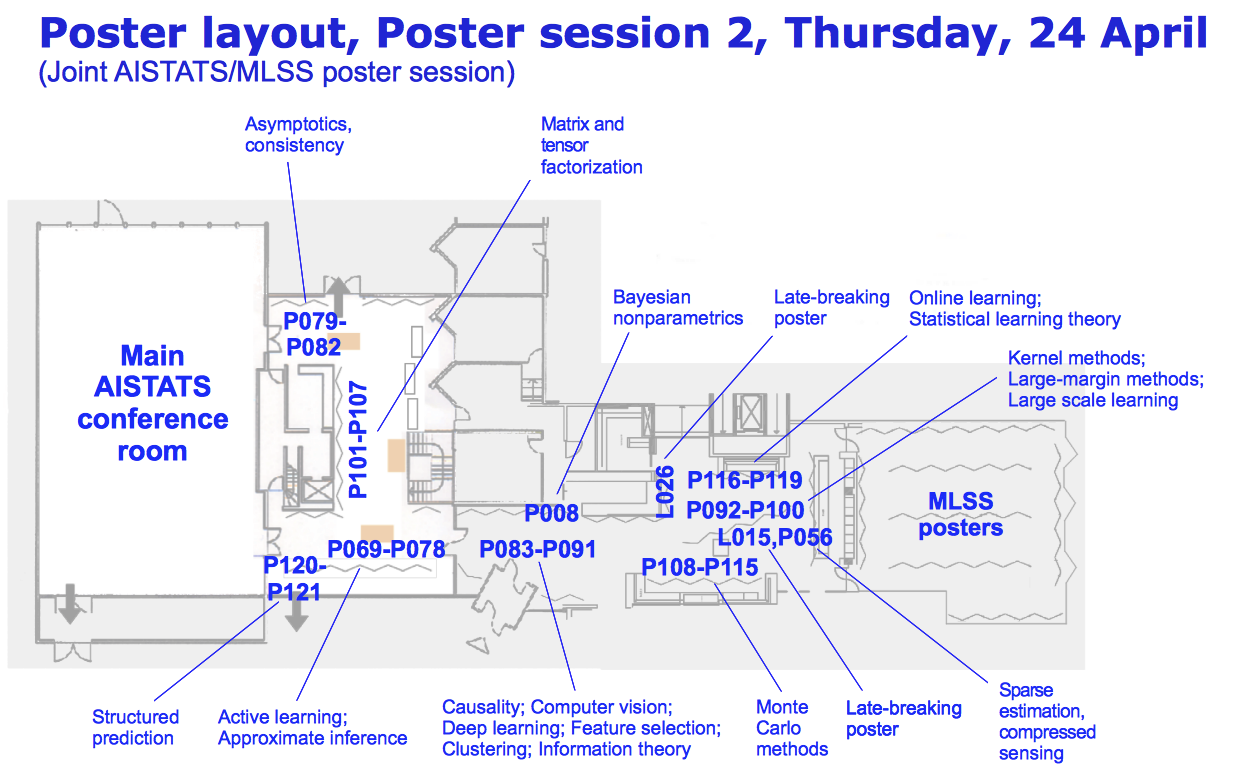
Click the image to view the layout in larger size.
Active learning
P069 Characterizing EVOI-Sufficient k-Response Query Sets in Decision Problems
Robert Cohn; Satinder Singh; Edmund Durfee
P070 Active Area Search via Bayesian Quadrature
Yifei Ma; Roman Garnett; Jeff Schneider
P071 Bayesian Multi-Scale Optimistic Optimization
Ziyu Wang; Babak Shakibi; Lin Jin; Nando de Freitas
P072 On correlation and budget constraints in model-based bandit optimization with application to automatic machine learning
Matthew Hoffman; Bobak Shahriari; Nando de Freitas
P073 Near Optimal Bayesian Active Learning for Decision Making
Shervin Javdani; Yuxin Chen; Amin Karbasi; Andreas Krause; Drew Bagnell; Siddhartha Srinivasa
P074 An Analysis of Active Learning with Uniform Feature Noise
Aaditya Ramdas; Barnabas Poczos; Aarti Singh; Larry Wasserman
Approximate inference
P075 Loopy Belief Propagation in the Presence of Determinism
David Smith; Vibhav Gogate
P076 Black Box Variational Inference
Rajesh Ranganath; Sean Gerrish; David Blei
P077 Expectation Propagation for Likelihoods Depending on an Inner Product of Two Multivariate Random Variables
Tomi Peltola; Pasi Jylänki; Aki Vehtari
P078 Efficient Lifting of MAP LP Relaxations Using k-Locality
Martin Mladenov; Kristian Kersting; Amir Globerson
Asymptotics, consistency
P079 Sparsity and the truncated l^2-norm
Lee Dicker
P080 Non-Asymptotic Analysis of Relational Learning with One Network
Peng He; Changshui Zhang
P081 Fast Distribution To Real Regression
Junier Oliva; Willie Neiswanger; Barnabas Poczos; Jeff Schneider; Eric Xing
P082 Gaussian Copula Precision Estimation with Missing Values
Huahua Wang; Farideh Fazayeli; Soumyadeep chatterjee; Arindam Banerjee
Causality
P083 On Estimating Causal Effects based on Supplemental Variables
Takahiro Hayashi; Manabu Kuroki
Computer vision
P084 Visual Boundary Prediction: A Deep Neural Prediction Network and Quality Dissection
Jyri Kivinen; Chris Williams; Nicolas Heess
Deep learning
P085 Avoiding pathologies in very deep networks
David Duvenaud; Oren Rippel; Ryan Adams; Zoubin Ghahramani
P086 To go deep or wide in learning?
Gaurav Pandey; Ambedkar Dukkipati
Feature selection and clustering
P087 An LP for Sequential Learning Under Budgets
Joseph Wang; Kirill Trapeznikov; Venkatesh Saligrama
P088 Jointly Informative Feature Selection
Leonidas Lefakis; Francois Fleuret
P089 An Efficient Algorithm for Large Scale Compressive Feature Learning
Hristo Paskov; John Mitchell; Trevor Hastie
P090 Cluster Canonical Correlation Analysis
Nikhil Rasiwasia; Dhruv Mahajan; Vijay Mahadevan; Gaurav Aggarwal
Information theory
P091 Bias Reduction and Metric Learning for Nearest-Neighbor Estimation of Kullback-Leibler Divergence
Yung-Kyun Noh; Masashi Sugiyama; Song Liu; Marthinus C. du Plessis; Frank Chongwoo Park; Daniel D. Lee
Kernel methods
P092 Learning and Evaluation in Presence of Non-i.i.d. Label Noise
Nico Görnitz; Anne Porbadnigk; Alexander Binder; Claudia Sannelli; Mikio Braun; Klaus-Robert Mueller; Marius Kloft
P093 Efficient Algorithms and Error Analysis for the Modified Nystrom Method
Shusen Wang; Zhihua Zhang
P094 A Geometric Algorithm for Scalable Multiple Kernel Learning
John Moeller; Parasaran Raman; Suresh Venkatasubramanian; Avishek Saha
P095 Recovering Distributions from Gaussian RKHS Embeddings
Motonobu Kanagawa; Kenji Fukumizu
Large margin methods
P096 A New Perspective on Learning Linear Separators with Large L_q L_p Margins
Maria-Florina Balcan; Christopher Berlind
Large scale learning
P097 In Defense of Minhash over Simhash
Anshumali Shrivastava; Ping Li
P098 Online Passive-Aggressive Algorithms for Non-Negative Matrix Factorization and Completion
Mathieu Blondel; Yotaro Kubo; Ueda Naonori
P099 Fugue: Slow-Worker-Agnostic Distributed Learning for Big Models on Big Data
Abhimanu Kumar; Alex Beutel; Qirong Ho; Eric Xing
P100 Scaling Graph-based Semi Supervised Learning to Large Number of Labels Using Count-Min Sketch
Partha Talukdar; William Cohen
Matrix and tensor factorization
P101 Efficient Distributed Topic Modeling with Provable Guarantees
Weicong Ding; Mohammad Rohban; Prakash Ishwar; Venkatesh Saligrama
P102 Robust Stochastic Principal Component Analysis
John Goes; Teng Zhang; Raman Arora; Gilad Lerman
P103 Scalable Collaborative Bayesian Preference Learning
Mohammad Emtiyaz Khan; Young Jun Ko; Matthias Seeger
P104 Latent Gaussian Models for Topic Modeling
Changwei Hu; Eunsu Ryu; David Carlson; Yingjian Wang; Lawrence Carin
P105 Scalable Variational Bayesian Matrix Factorization with Side Information
Yong-Deok Kim; Seungjin Choi
P106 Collaborative Ranking for Local Preferences
Berk Kapicioglu; David Rosenberg; Robert Schapire; Tony Jebara
P107 Estimating Dependency Structures for non-Gaussian Components with Linear and Energy Correlations
Hiroaki Sasaki; Michael Gutmann; Hayaru Shouno; Aapo Hyvarinen
Bayesian nonparametrics
P008 A Non-parametric Conditional Factor Regression Model for Multi-Dimensional Input and Response
Ava Bargi; Richard Yi Xu; Zoubin Ghahramani; Massimo Piccardi
Monte Carlo methods
P108 LAMORE: A Stable, Scalable Approach to Latent Vector Autoregressive Modeling of Categorical Time Series
Yubin Park; Carlos Carvalho; Joydeep Ghosh
P109 Accelerating ABC methods using Gaussian processes
Richard Wilkinson
P110 Scaling Nonparametric Bayesian Inference via Subsample-Annealing
Fritz Obermeyer; Jonathan Glidden; Eric Jonas
P111 Generating Efficient MCMC Kernels from Probabilistic Programs
Lingfeng Yang; Patrick Hanrahan; Noah Goodman
P112 Approximate Slice Sampling for Bayesian Posterior Inference
Christopher DuBois; Anoop Korattikara; Max Welling; Padhraic Smyth
P113 A Level-set Hit-and-run Sampler for Quasi-Concave Distributions
Shane Jensen; Dean Foster
P114 A New Approach to Probabilistic Programming Inference
Frank Wood; Jan Willem van de Meent; Vikash Mansinghka
P115 Spoofing Large Probability Mass Functions to Improve Sampling Times and Reduce Memory Costs
Jon Parker; Hans Engler
Online learning and statistical learning theory
P116 Robust Forward Algorithms via PAC-Bayes and Laplace Distributions
Asaf Noy; Koby Crammer
P117 Doubly Aggressive Selective Sampling Algorithms for Classification
Koby Crammer
P118 Selective Sampling with Drift
Edward Moroshko; Koby Crammer
P119 Improved Bounds for Online Learning Over the Permutahedron and Other Ranking Polytopes
Nir Ailon
Sparse estimation, compressed sensing
P056 Algebraic Reconstruction Bounds and Explicit Inversion for Phase Retrieval at the Identifiability Threshold
Franz Király; Martin Ehler
Structured prediction
P120 Efficiently Enforcing Diversity in Multi-Output Structured Prediction
Abner Guzman-Rivera; Pushmeet Kohli; Dhruv Batra, Virginia Tech; Rob Rutenbar
P121 Learning Structured Models with the AUC Loss and Its Generalizations
Nir Rosenfeld; Ofer Meshi; Danny Tarlow; Amir Globerson
Late-breaking Posters
L015 Comparing Binary Hamiltonian Monte Carlo and Gibbs Sampling for Training Discrete MRFs with Stochastic ApproximationHanchen Xiong; Sandor Szedmak; Justus Piater
L026 Asymmetric Clustering Index for partitions validation
Marek Śmieja; Dawid Warszycki; Jacek Tabor; Andrzej Bojarski
Additionally, MLSS posters will be shown in the Thursday poster session.